By Matthew Waller & Jerry Louis-Jeune
You know how we’ve all dreamed of having a crystal ball to see into the future? Well, businesses are no different. They’ve always wanted to know what’s coming next, and that’s where predictive analytics comes in. But here’s the catch: not everyone is a data whiz, and setting up the tech for predictive analytics can be like assembling furniture without instructions. That’s where Machine Learning as a Service (MLaaS) becomes a lifesaver. Think of it as the easy button for harnessing the future-predicting powers of data.
In this article, we’re diving into the world of MLaaS, the tool that’s making complicated data stuff accessible for regular folks. It’s changing the game for how businesses understand their customers, make decisions, and even see into the future. So, whether you’re a business owner, a curious techie, or someone who just likes staying on top of trends, stick around!
What is Machine Learning?
Machine learning (ML) is the process of teaching computers to learn from data and make predictions or decisions without explicit programming. ML has become a powerful tool for solving complex problems and unlocking new opportunities in various domains. However, developing and deploying ML solutions can be challenging, time-consuming, and costly, especially for small and medium-sized businesses (SMBs) that lack the resources and expertise to do so.
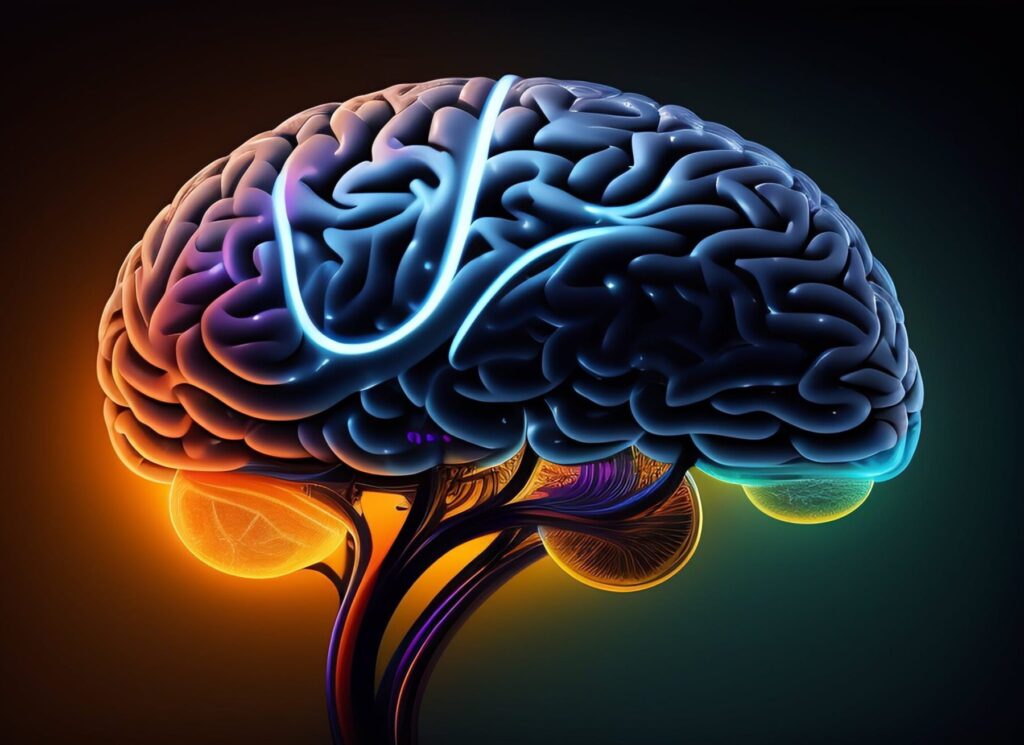
The Difference Between Machine Learning and Artificial Intelligence
While machine learning and artificial intelligence (AI) are often used interchangeably, they are not the same. AI is a broader concept that refers to machines or software possessing the ability to perform tasks that would typically require human intelligence—like problem-solving, understanding natural language, and visual perception. Machine learning is a subset of AI that focuses on the development of algorithms that can learn from data.
The Genesis and Evolution of Machine Learning as a Service
Emerging as a natural progression from the Software as a Service (SaaS) model, “Machine Learning as a Service” (MLaaS) takes the concept of software accessibility to a new frontier. The SaaS model revolutionized software deployment by allowing users to access applications via the internet, without worrying about infrastructure and maintenance. MLaaS extends this revolution to the realm of machine learning. It integrates machine learning algorithms and tools into cloud services, thereby simplifying and democratizing the use of complex machine learning technologies across a range of sectors.
In this new paradigm, MLaaS doesn’t merely offer cloud-based computing resources; it delivers actionable predictive analytics solutions. With MLaaS, users can readily tap into pre-trained machine learning models or even develop custom models, all accessible through cloud platforms, Application Programming Interfaces (APIs), or web applications. This eliminates the need for specialized coding skills or in-house infrastructure, making machine learning more accessible and user-friendly than ever before.
What Exactly is Machine Learning as a Service? MLaaS Definition
Machine Learning as a Service (MLaaS) is essentially a comprehensive suite of services integrated into cloud computing platforms. This includes an assortment of machine learning tools ranging from data visualization and facial recognition to natural language processing and beyond. By hosting these services on the cloud, MLaaS providers handle all the backend complexities, liberating businesses to implement machine learning solutions effortlessly, without the burden of requiring specialized in-house skill sets.
What Does MLaaS Imply for Businesses and Individuals?
The advent of MLaaS democratizes access to machine learning. No longer do you need a team of data scientists and a large budget to leverage machine learning. Businesses can now plug into machine learning algorithms to analyze data and make decisions in real-time. This brings about efficiencies, reduces costs, and can provide a competitive advantage.
For individuals, MLaaS offers a chance to integrate advanced analytics into their daily lives. Whether it’s a healthcare app that monitors vital stats or an educational platform that adapts to each learner, the applications are practically limitless.
Advantages of Machine Learning as a Service (MLaaS) over traditional machine learning
MLaaS offers several advantages over traditional machine learning, including:
Reduced costs
MLaaS eliminates the need for expensive hardware, software, and maintenance, as well as the hiring and training of data scientists and engineers. Users only pay for what they use, and can scale up or down as needed.
MLaaS reduces cost in several ways:
- It eliminates the need for upfront capital expenditure on infrastructure and licenses.
- It allows for flexible and scalable usage of resources based on demand and performance.
- It reduces the operational and maintenance costs associated with managing and updating the systems.
- It provides access to a variety of pre-built models and algorithms that can be customized and deployed quickly.
- It enables faster and easier integration of machine learning solutions with existing data sources and applications.
Increased efficiency
MLaaS allows users to quickly and easily deploy, scale, and update machine learning models, as well as integrate them with existing workflows and systems. Users can also leverage the cloud’s computing power and storage capacity to handle large and complex data sets.
Deployment: MLaaS enables users to deploy their machine learning models in minutes, without having to worry about setting up servers, installing libraries, or configuring environments. Users can simply upload their data and models to the cloud platform of their choice, and use a web interface or an API to access them. This saves time and resources that can be used for other tasks.
Scalability: MLaaS also allows users to scale their machine learning models according to their needs, without having to worry about performance issues or capacity limits. Users can easily adjust the number of instances, the type of hardware, or the amount of data that their models use, and pay only for what they use. This ensures that users can handle any volume or complexity of data, and deliver fast and accurate results.
Integration: MLaaS also facilitates the integration of machine learning models with existing workflows and systems. Users can easily connect their models to various data sources, such as databases, APIs, or web services, and use them for various purposes, such as prediction, classification, recommendation, or analysis. Users can also embed their models into applications, dashboards, or reports, and share them with other users or stakeholders. This enhances the value and usability of machine learning models.
Improved accuracy
One of the benefits of MLaaS is that it improves accuracy by leveraging the latest advances in machine learning algorithms, data quality, and security, as well as the collective intelligence of the cloud, to deliver more accurate and reliable predictions. Users can also benefit from the feedback and improvement of other users who use the same or similar models.
Some of the ways that MLaaS can improve accuracy are:
- Data preprocessing: MLaaS platforms can offer data cleaning, transformation, and feature engineering services that can help users prepare their data for machine learning. This can reduce noise, outliers, missing values, and other issues that can affect the performance of models.
- Model selection: MLaaS platforms can offer a variety of machine learning algorithms and frameworks that can suit different use cases and objectives. Users can choose from pre-trained models or customize their own models using various parameters and hyperparameters. This can help users find the best model for their data and problem.
- Model validation: MLaaS platforms can offer tools and metrics to evaluate the performance of machine learning models on different datasets. Users can test their models on training, validation, and test sets, and compare them with baseline models or benchmarks. This can help users identify and correct errors, biases, and overfitting issues.
- Model deployment: MLaaS platforms can offer scalable and secure infrastructure to deploy machine learning models as APIs or web applications. Users can integrate their models with other systems and platforms, and monitor their performance and feedback in real-time. This can help users update and improve their models based on new data and user behavior.
Faster time to market
One of the main benefits of MLaaS is that it allows for faster time to market. This means that users can leverage the existing expertise and resources of the MLaaS providers to accelerate the development and deployment of their machine learning solutions. Some of the ways that MLaaS enables faster time to market are:
- Pre-built models and frameworks: MLaaS providers offer a range of pre-built models and frameworks that cover common use cases and scenarios, such as image recognition, natural language processing, sentiment analysis, etc. Users can easily access and customize these models and frameworks to suit their specific needs, without having to start from scratch or reinvent the wheel.
- Scalable and elastic infrastructure: MLaaS providers offer scalable and elastic infrastructure that can handle large volumes of data and compute-intensive tasks. Users can easily scale up or down their machine learning workloads according to their demand, without having to worry about provisioning, managing, or maintaining servers, storage, or network.
- Automated and simplified workflows: MLaaS providers offer automated and simplified workflows that streamline the entire machine learning lifecycle, from data ingestion and pre-processing to model training and testing, to deployment and monitoring. Users can focus on the core logic and business value of their machine-learning solutions, without having to deal with the technical complexities and challenges of each stage.
- Collaboration and integration: MLaaS providers offer collaboration and integration features that enable users to work together and share their machine learning projects with other stakeholders. Users can also integrate their machine learning solutions with other cloud-based services and applications, such as data sources, analytics tools, visualization tools, etc., to create end-to-end solutions.
MLaaS has a wide range of applications across various industries, such as high-tech, healthcare, finance, retail, education, and more. For example, MLaaS can help in these areas.
Diagnose diseases, recommend treatments, and monitor patients’ health
One of the promising applications of MLaaS is in the healthcare sector, where machine learning can help to diagnose diseases, recommend treatments, and monitor patients’ health. Here are some examples of how MLaaS can benefit healthcare:
- Diagnose diseases: MLaaS can enable faster and more accurate diagnosis of various diseases by analyzing medical images, lab tests, symptoms, and other data. For instance, MLaaS platforms can provide computer vision services that can detect anomalies in X-rays, CT scans, MRI scans, or mammograms. MLaaS platforms can also provide natural language processing services that can extract relevant information from medical records, clinical notes, or patient feedback.
- Recommend treatments: MLaaS can help to personalize and optimize treatments for different patients by taking into account their medical history, preferences, allergies, and other factors. For example, MLaaS platforms can provide recommendation systems that can suggest the best drugs, dosages, or therapies for each patient. MLaaS platforms can also provide reinforcement learning services that can learn from the outcomes of previous treatments and adjust the recommendations accordingly.
- Monitor patients’ health: MLaaS can help to track and improve patients’ health by collecting and analyzing data from wearable devices, mobile apps, or sensors. For example, MLaaS platforms can provide predictive analytics services that can identify potential risks or complications before they become serious. MLaaS platforms can also provide sentiment analysis services that can measure patients’ emotions, moods, or satisfaction levels.
Detect fraud, optimize pricing, and personalize customer service
MLaaS can help financial institutions and businesses in a number of areas. It can:
- Detect fraud: Fraud is a serious threat for many businesses, especially in the financial sector. Fraudsters use sophisticated techniques to deceive customers, steal identities, and manipulate transactions. MLaaS can help to detect fraud by analyzing large volumes of data and identifying patterns, anomalies, and behaviors that indicate fraudulent activity. For example, MLaaS can help to detect credit card fraud by comparing each transaction with the customer’s historical data and flagging any unusual or suspicious ones. MLaaS can also help to prevent fraud by providing real-time alerts and recommendations to customers and employees.
- Optimize pricing: Pricing is a key factor that influences customer demand, revenue, and profit. However, finding the optimal price for each product or service can be challenging, as it depends on various factors such as market conditions, customer preferences, competitors’ actions, and costs. MLaaS can help to optimize pricing by using data-driven algorithms that can dynamically adjust prices based on supply and demand, customer segmentation, and other variables. For example, MLaaS can help to optimize pricing for e-commerce platforms by analyzing customer behavior, purchase history, and browsing patterns and offering personalized discounts or incentives.
- Personalize customer service: Customer service is an essential aspect of customer satisfaction and loyalty. However, providing high-quality customer service can be costly and time-consuming, especially when dealing with large numbers of customers with diverse needs and expectations. MLaaS can help to personalize customer service by using natural language processing (NLP) and chatbots that can understand customer queries, provide relevant answers, and offer tailored solutions. For example, MLaaS can help to personalize customer service for travel agencies by using chatbots that can recommend destinations, flights, hotels, and activities based on customer preferences and budget.
Forecast demand, optimize inventory, and enhance customer loyalty
MLaaS can also help businesses forecast demand, optimize inventory, and enhance customer loyalty by providing them with scalable, flexible, and cost-effective machine learning solutions.
One of the applications of MLaaS is to forecast demand, optimize inventory, and enhance customer loyalty. Demand forecasting is a crucial part of all businesses, as it helps them plan their production, pricing, inventory, and logistics. However, traditional methods of demand forecasting are often inaccurate, inefficient, and time-consuming.
Machine learning can improve demand forecasting by using historical data and external factors, such as weather, economic trends, and customer behavior, to predict future demand patterns. Machine learning can also handle complex and dynamic data sets, such as seasonal variations, product life cycles, and promotions. Machine learning can provide accurate and timely forecasts that can help businesses reduce costs, increase revenue, and improve customer satisfaction.
Inventory optimization is another benefit of MLaaS. Inventory optimization is the process of finding the optimal balance between inventory levels and customer demand. Too much inventory can lead to waste, obsolescence, and storage costs. Too little inventory can lead to stockouts, lost sales, and customer dissatisfaction.
Machine learning can help optimize inventory by using demand forecasts and other data sources to determine the optimal inventory levels for each product, location, and time period. Machine learning can also account for uncertainties, such as supply chain disruptions, demand fluctuations, and lead times. Machine learning can help businesses minimize inventory costs, maximize service levels, and increase profitability.
Customer loyalty is a key factor for business success. Customer loyalty is the degree to which customers are satisfied with a business’s products or services and are willing to repeat purchases or recommend them to others. Customer loyalty can lead to increased retention, referrals, cross-selling, and upselling.
Machine learning can help enhance customer loyalty by using data to understand customer preferences, needs, and behavior. Machine learning can also segment customers based on their value, loyalty, and potential. Machine learning can help businesses personalize their offerings, communications, and interactions with each customer segment. Machine learning can help businesses increase customer satisfaction, engagement, and loyalty.
Assess students’ learning, provide feedback, and recommend courses
One of the main applications of MLaaS in education is to assess students’ learning outcomes and progress. MLaaS can use data from various sources, such as quizzes, assignments, surveys, and online activities, to analyze students’ performance, strengths, weaknesses, and preferences. MLaaS can also use natural language processing (NLP) to evaluate students’ written and spoken responses, and computer vision to recognize students’ gestures and expressions. By using MLaaS, teachers can get a comprehensive and objective view of students’ learning and tailor their instruction accordingly.
Another application of MLaaS in education is to provide feedback to students and teachers. MLaaS can use artificial intelligence (AI) to generate personalized and timely feedback for students based on their learning goals, progress, and challenges. MLaaS can also use sentiment analysis to detect students’ emotions and motivation levels and provide appropriate encouragement and support. For teachers, MLaaS can provide feedback on their teaching methods, strategies, and effectiveness, and suggest ways to improve their pedagogy.
A third application of MLaaS in education is to recommend courses and learning resources for students. MLaaS can use recommender systems to suggest courses and materials that match students’ interests, needs, and abilities. MLaaS can also use collaborative filtering to recommend courses and resources that are popular or relevant among similar or connected students. By using MLaaS, students can discover new learning opportunities and expand their knowledge and skills.
And much more!
MLaaS is a game-changer for predictive analytics, as it democratizes access to machine learning and enables users to harness its power without compromising on quality, speed, or cost.
MLaaS by Sapientai: A Beacon in the World of Predictive Analytics
When it comes to offering next-level machine learning solutions, Sapientai leads the pack. Our MLaaS platform is not just another tool in the shed; it’s a comprehensive ecosystem built to serve various industries, from healthcare and finance to cutting-edge scientific research like nuclear fusion. We’re not just providing a service; we’re revolutionizing predictive analytics. Here’s how:
The Sapientai Edge
- Extensive Library of Models: Users have instant access to a library teeming with ready-to-use machine-learning models. Whether you’re looking to nail sentiment analysis, perfect image recognition, or anything in-between, we’ve got you covered.
- DIY Custom Models: Create your machine learning models with ease using our intuitive drag-and-drop interface. For the tech-savvy, uploading your own data and code is a breeze.
- Flexible Deployment: Choose where you want your models to live. Our platform supports both cloud and on-premise deployments.
- MLOps Services: Our robust MLOps solutions ensure that your machine learning models aren’t just built but are continually optimized for peak performance, accuracy, and security.
Partnerships that Speak Volumes
Our success is amplified by our strategic partnerships across multiple sectors. We’ve teamed up with industry leaders like General Atomics, HPE, ARPA, General Fusion, and have been instrumental in projects that aim to tackle big problems—like working on nuclear fusion for sustainable energy solutions.
Customized and Scalable Solutions
We get it; one size doesn’t fit all. That’s why our MLaaS services are tailored to meet the unique needs of each user. Whether you’re a startup taking your first steps or a Fortune 500 company, our platform scales to meet your needs without breaking the bank.